Summary
In this session, Steve Horvath, professor at the University of California Los Angeles, provided an overview of the current state of the epigenetic clock field and the new developments in it. Then he went on to talk about what is missing in regards to methylation clocks and the longevity field itself, as well as what might be the next steps – the holy grails we should strive to get to. He also went to address some of the common misconceptions tied to epigenetic clocks at the end.
Presenters
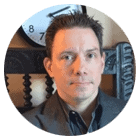
Steve Horvath, UCLA
I am a Professor in Human Genetics and Biostatistics at UCLA. My methodological research area lies at the intersection of biostatistics, bioinformatics, computational biology, cancer research, genetics, epidemiology, machine learning, and systems biology. My group applies these methods to study a broad spectrum of diseases, e.g. aging research, cancer, cardiovascular disease, HIV, Huntington’s disease, neurodegenerative diseases….
- What’s new and what’s missing?
- In this talk the meaning of clock is as an algorithm, a number, a biomarker.
I have developed a pan-tissue clock that applies to all human cells and tissues. But there is a question. Is blood a good surrogate tissue for other organs? Is it sufficient to just measure blood methylation? I generated here methylation data from roughly 120 postmortem individuals for whom we have measured different tissues (blood, liver, spleen, bone marrow, and so on). From which we can define this measure of epigenetic age acceleration as a residual of methylation age where you regress out chronological age. And you can ask what’s the correlation of epigenetic age acceleration in blood vs. in bone marrow, and it’s a high correlation. But then you see a surprising result in the liver. Correlation of blood and liver age acceleration in methylation is negligible. You can really see this analysis as a glass half full or half empty way. Either the current clocks show pretty well conservation across tissue types, or maybe blood age acceleration is not really related to heart age acceleration or liver. So there is an opportunity to develop new human biomarkers that really hone in on cytosines that are conserved. Whose aging effects are conserved across tissues. It would be great to have one source of DNA, where you could capture and measure the epigenetic age of all others. The extreme example is the brain, we all want to measure the brain, but what accessible tissue can serve as a surrogate?
I wanted to show you a surprising effect from a different study. The upper row shows the effect of hypertension. Hypertension was associated with age-acceleration in different tissues, but not all organs. It could be that certain age-related diseases will affect epigenetic aging in many organs while others might have only a precise effect on one specific organ. So if you want to predict mortality, is blood methylation sufficient? Or should you measure methylation in multiple tissues and cell types?
Moving on to another topic. Many of you may know that we developed a new array for measuring methylation in all mammalian species. It can be purchased through my non-profit – Epigenetic Clock Development Foundation. If you are interested, reach out to Bobby Brooke – it’s a non-profit so we want to promote the use of clocks.
The array was designed to tolerate mutations across species. Some of the nucleotides in different species are mutated. But if you still want to measure them, you need to have oligonucleotide probes that still bind, and that’s very much done by this array.
We built very accurate clocks for the naked mole rat, an animal that has negligible senescence. But according to epigenetic clocks it definitely ages.
Another application of clocks. This is a dual species clock. You can take this one regression model that measures relative age in both humans and rats. The orange dots correspond to rat samples and the green dots to human samples, and you can see they overlap and overlay. That’s because we defined the relative age (x axis) as chronological age in units of years divided by the maximum lifespan of the species, so it is normalized. And it is just one of these miracles of cytosine methylation that you can build very accurate dual species clocks.
We didn’t stop there and aimed to develop an universal mammalian clock (in hindsight a better name would be pan-mammalian clock). This is one regression model for measuring relative age across all mammalian species.
This is a result of application to over 10,000 tissue samples, labeled by species (over 150 species). And you see the methylation levels predict relative age with the correlation close to 0.95. It is a miracle that this is possible. Why? We are using the maximum lifespan of the species in the denominator, but this maximum lifespan can often only be estimated (think of the debate about the maximum to human lifespan), but even in spite of that substantial noise, methylation allows us to get this very accurate and substantial correlation. And it is even reassuring that even in the worst case (application of pan-mammalian clock to marsupials), this universal clock still works.
This is how the universal clock works in specific species, as you can see it works very well in most species.
Different topic – forget chronological age. If we want to figure out maximum lifespan (a fundamental life history trait), can you predict it based on methylation? And the answer is clear, we can see a correlation of almost 0.9, so this is a rare profound insight that methylation allow us to get.
We have this estimate of the maximum lifespan, now the question is what about growth hormone knockout mice? Their lifespan is very much increased compared to regular mice. And interestingly, this panel shows you that this maximum lifespan predictor shows this expected association, the knockout mice have a predicted maximum lifespan that’s older than the normal mice. The right panel then shows caloric restriction for comparison, and there is no effect. We all know that CR changes at least the average maximum lifespan of the mice, no question, but the question is does it really change the species characteristic? According to this analysis, it does not. It is very important to establish species characteristics such as maximum lifespan from what people often care about, which is the risk estimate of mortality or morbidity. Lots of things relate to mortality but they may not change the maximum lifespan of the species.
Different topic. This is always the quote that I think when we have speakers here from the FDA and we talk about biomarkers. Yes, in 20 years we will have amazing biomarkers, but we need some solutions right now that can be deployed today. Do we have one? Kind of.
GrimAge, I am quite optimistic about it. I am aware of its many limitations, more than anyone else. However it does have its strengths. There is a very strong literature support for mortality predictions in relation to many age-related diseases, validated by independent groups. Also people started doing longitudinal studies where they relate the rate of change of GrimAge with increased hazard of death, with encouraging results, which might indicate causality. There are also large genetic studies, where we developed polygenic risk scores which were associated with parental longevity. This is important because epidemiologists often use genetic arguments to argue causality. And GrimAge performs exceptionally well when talking about analytical validation. GrimAge has exceptionally high intraclass correlation in all BLOOD data sets. The good news is that we have things we can work with, these are first versions, and we continually develop new versions that improve over the previous versions.
Challenges particularly in the biomarker field, which will be more of a philosophical discussion.
Let’s start with the fundamental challenge of biological age. The problem is that mathematically speaking it is a latent variable, we don’t have a definition, you need to infer it from manifest variables (actual variables). We can use these molecular readouts (omics) to infer it, conversely pathologists would look at cellular changes, tissue pathology, then there are clinical readouts like frailty, and so on. Our field of aging biomarkers needs to find a way to integrate this information. We have one good starting point – the chronological age. Nobody ever debates that biological age increases with chronological age. So we have a starting point. What does it mean? If you have a biomarker that does not correlate with chronological age, you have a problem (think viral load, might predict mortality but is not tied to aging). Personally I am interested in biomarkers that measure innate aging processes.
Here is the challenge in the aging field. There is really no consensus on an established theory of aging. We could spend two days debating very plausible theories of aging, but there is no consensus. Contrast this to the cancer field, where they have well established oncogenes, tumor suppressor, they understand pathways, treatments, and suddenly their theory is far more developed. Then there is another lack of consensus, if you don’t have a theory, you really have a hard time defining biomarkers of aging. So we really don’t have any consensus on proxy endpoints of aging. Again if we contrast it with other medical fields, we clearly have wonderful biomarkers for example for anti-hypertensive medication.
How do we arrive at a consensus in the aging field? There is a medieval solution, developed by the catholic church.
This is what would probably happen if we tried that in the aging field, so we need a different solution.
I believe capitalism will solve this problem. Companies that use effective biomarkers will succeed at developing effective anti-aging interventions. Success will ultimately lead to emulation and adoption.
Now about some open and closed goals of the aging field. I always thought that the old holy grail are aging clocks that apply to all tissues and cell types. In my world, aging happens in most tissues and cell types, so biomarkers should measure aging in all of these tissues and cell types. Then there is another holy grail – we all believe that aging applies to all mammals, so it would be good to have aging clocks that apply to all mammalian tissues, not just humans. The status of these old holy grails is that we have reasonably good solutions based on cytosine methylation. If you contrast that with biomarkers based on transcriptomic data, this will be rough. However my hope is that the field will find other readouts, because cytosine methylation is limited, we need markers of autophagy, mitochondrial function, stem cell function, senescent markers, all of that. So there are still opportunities to address these old goals with new data perhaps.
- Now about the new holy grails. The first is clearly single cell clocks – to deal with confounding by cell type composition. Bulk tissue used for current clocks comprises of many different cell types and we would like to know what’s the age of a stem cell or a more differentiated cell. We also need the single cell clocks for mechanistic understanding of the aging process, maybe they could help us get closer to a theory of aging. And they may also explain how molecular changes relate to cellular phenotypes and ultimately tissue dysfunction. In the slide is a roadmap of clocks we need to build up starting from specific cell type level of complexity up to a clock working for mammalian cell types. This is a very exciting area, ongoing status.
- The other holy new (or maybe unsolved old) grail is a causal clock. Could be based on bulk tissues, the problem is people don’t have a good definition of them.
- What is meant by it? If you reverse the age estimates of the causal clock, it will convey health benefits to a patient. Fundamentally that’s what people want, if you rejuvenate by 10 years, you would like them to live 10 years longer. Some people say mechanistic understanding is mandatory, but I would be happy if somebody uses some AI and develops a blackbox biomarker that meets the above criteria.
- The question is, where are we with these clocks? Well, we have to distinguish between species. When it comes to mice, we are almost there, we have many clocks that respond to interventions like CR, GH KO, parabiosis, or conversely high fat diet. I’m a bit on the fence about rapamycin with regards to the epigenetic clock – I saw datasets and clocks that worked and that didn’t. We need more data. But with regards to mice, overall we almost have it.
However, we of course want a causal clock for humans. And there we face this fundamental problem, that we really don’t have validated anti-aging interventions in humans. Without proxy endpoints we cannot validate interventions in humans. And conversely, without interventions we cannot validate the proxy endpoints and biomarkers. We want to test the test, and if we don’t have gold standard interventions in humans, we have a problem. There are a few workarounds listed in the slide I can think of.
People always ask me these questions, so I’ll answer a few of them upfront. Epigenetic changes are causal, but we don’t know to what extent, and in which tissue, and there are different ways to define epigenetic ages. So there is a lot to work out, but the answer to yes/no question is yes.
Now comes another question. Is cytosine methylation causal? Yes and no, ambiguous. You can find plenty of examples to support both the points of view. There are powerful experiments that argue for causal roles for methylation. But also powerful arguments for no – C. elegans doesn’t have cytosine methylation, but C. elegans ages. So if you make the universal assumption that aging is universally conserved between invertebrates and vertebrates, you have a problem. And also many cytosines gain or lose methylation and it doesn’t affect neighboring gene expression, so the transcriptomic consequences are often entirely unclear. Some changes will be causal and some will not be. The challenge of the field is to develop clocks that hone in on these causal changes. We need comprehensive studies between different omics, interventional studies, etc.
There were some conversations about cell composition confounding methylation clocks. The problem is it can confound almost any omics data. Changes of cell composition can actually be part of the signal. If you ask what is the cellular manifestation of epigenetic ages, well maybe if you change the epigenome, it changes the stem cell function and leads to changes in cell composition – maybe it can be a part of the causal chain. This is in my mind a selling point for pan-tissue clocks, because they are overall quite robust to changes in cellular composition.
Probably a very complex network with many network effects, we need more research.
Q&A
What can this group do to help you in your endeavor?
- Non-profit foundation – if you have an exciting study, please contact us. We are looking for exciting interventional studies, because they help us characterize the clocks.
- Many of you work on the regulatory aspects, engaging with the FDA, I am grateful for that.
How come you don’t mention senolytics in relation to epigenetic clocks?
- I don’t really have data about senolytics – if somebody has a senolytics study, email me. Or if you know about anybody who has, ping any of the people who have the data.
For your species maximum lifespan clock, isn’t it possible that they reflect body sizes?
- These relationships between cytosine methylation and maximum lifespans are not according to body sizes, we looked at that. In terms of growth, I don’t think so as well.
I’m wondering about what exactly your clocks are measuring – are there specific sites shared across mammalian species, or could I take random CpGs and predict age/lifespan with those?
- Multiple answers. We have one cytosine that correlates very well with age. But it would probably be a bad clock by itself, so then you get a selection of cytosines that are correlated better together. They are not random, there is biology behind them, there is definitely a biological insight.
- Another question is whether you can pick random 5000 cytosines and build a clock? Most likely yes, because they would cover most of the cytosines that are the most correlated. Most of our clocks use hundreds, but you could probably pick different ones to get good results.
You mentioned that mice under caloric restriction do not have significant changes in their epigenetic clock, but studies have shown only some mice strains will have an enhanced lifespan under caloric restriction (e.g. this). Have you compared clocks between different strains that have different lifespans or healthspans?
- CR slows epigenetic clocks. What I showed you was an estimate of maximum lifespan, where we didn’t see the effect, so I don’t know what to think about that.
I’ve been a skeptic of the significance of epigenetic clocks. However, this began changing with the in vivo reprogramming data. Now I see there is also a potential mechanism: double-strand-break repair. This is possibly a driver of age-related epigenetic damage and information loss. Do you think this mechanism is significant? Does it help inform the pursuit for a causal DNAm clock?
- I love the theory, David Sinclair has these wonderful experiments that argue it is a major mechanism, it looks very convincing. But I do have one concern – we did very detailed radiation experiments, that has been overall a failure. In vitro it didn’t lead to age acceleration, but in vivo we saw it. And the radiation wasn’t picked up by the epigenetic clock.
What is the connection between GrimAge and cigarettes? (historical interest).
- It definitely tracks smoking history, it uses that in its construction. That can be good or bad. If you feel that smoking increases biological age, you want it constructed based on that. If you think smoking is more of a stress response, you might not want it. In that case, the pan-tissue clock doesn’t relate to smoking at all. There are many clocks now, I am very happy about that. Clocks have different properties, so it’s important to keep that in mind.
What do you think would have to happen to aging clocks for the FDA to get interested?
- I have only a naive comment – finding a successful intervention that will change the world. We need this kind of positive example – the problem is that many of the discussions are very theoretical.
- I have high hopes for Greg Fahy and Intervene Immune – I look at it as an investor though – we need a broad portfolio of different interventions, and some of them might hit.
Can you talk a little bit more about your thoughts on developing multiple clocks for different omics modalities? I get the impression that having several (ideally single cell) clocks for eg. RNA, chromatic accessibility, methylation and proteomics would be a way to get around the issue of causality, because presumably if an intervention manages to affect all these clocks on separate modalities, then it would be affecting some of the underlying biology, and just not changing the particular signal that we are measuring.
- I agree, the more checkmarks you have, the better. One thing I will say is that if you are heavily involved in omics, systems biology, integromics, etc., you will learn that when you really analyze data, adding one more layer might obliterate the clock. So five different independent clocks would be the dream. It’s an obvious avenue, it’s more about convincing others to apply it. And combine the biomarkers. I actually started on transcriptomic clocks, but wasn’t successful at my time.
Seminar summary by Bolek Kerous.