Presenters
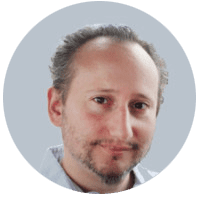
Richard Mallah, Future of Life Institute
Richard is Director of AI Projects at the Future of Life Institute, where he works to support the robust, safe, beneficent development of both short-term and long-term artificial intelligence via analysis, metaresearch, organization, research direction, and advocacy. Among his research interests at FLI are multiobjective ethical ensembles, semantic overlay of subsymbolic and neuromorphic processes, and dynamic roadmapping of the future. Mallah also heads research in ā¦
Georgios Kaissis, Technical University of Munich: Institute for Artificial Intelligence in Medicine
Georgios Kaissis is a senior research scientist at the Institute of Artificial Intelligence and Informatics in Medicine and specialist diagnostic radiologist at the Institute for Radiology at TUM, a postdoctoral researcher at the Department of Computing at Imperial College London and leads the Healthcare Unit at OpenMined. His research concentrates onā¦
Foresight Fellow Q&A:
Richard Mallah Q&A
Richard is an honorary Foresight fellow working on advanced AI safety, advanced knowledge learning, training agents, and more for 20+ years. He is concerned with general AI ā there needs to be a much richer understanding of good and bad to mitigate AI behavioral risk. He has experience in knowledge representation and knowledge learning, and has seen these fields evolve over time.
Q&A Takeaways
- Time is short, we need to act fast as the future approaches
- We need a portfolio approach to AI safety
- The end goal is to have AI and AGI turn out well for life
- Stepwise explainability can be used to refine robust article-writing AI
- Ontology restructuring will give us useful info
- There are conflicting human values and we cannot wait for consensus when building AI
- We need a principled approach for combining top down and bottom up approaches to ethics
- We should have confidence in a broad landscape of accessible safety methods
Georgis Kaissis Q&A
Georgis is an assistant professor at the Technical University of Munich, a research associate at the Imperial College in London, leads the research team at OpenMind ā a large open source collective ā and works on developing open source software. He is interested in privacy preserving machine learning, which is a trifecta of technology that allows us to derive information from data without seeing the data.
Q&A Takeaways
- There is a need for more data than ever before but this clashes with privacy
- Zero copy problem ā data diminishes in value the more you copy it
- No data sharing system in existence will solve the problem of data misuse
- In medical AI, there are lots of tools which are trained on very small datasets with poor distribution due to privacy concerns
- We need a class of techniques that derive critical insights from data without seeing the entire datasets
- Language AI could expose social security numbers or other sensitive information when trained on extremely large datasets
- Private machine learning could allow AI to train on extremely large datasets without allowing for violations of privacy
- Differential privacy allows people to deny participation in a group while simultaneously being in the group. It works by introducing a certain amount of noise into the results which does not modify the outcome but allows participants on an individual level to claim non-adherance.
- Data can also be split up and analyzed in pieces, then reconstituted later
- Complex valued weight models could be used to train AI in order to maintain privacy
- There are tradeoffs between generalization and memorization, and the nature of memorization and learning is being actively worked on
- Finding a universal ethical standard for privacy would be very challenging, its probably better to compartmentalize the ethics models based on jurisdiction