Presenter
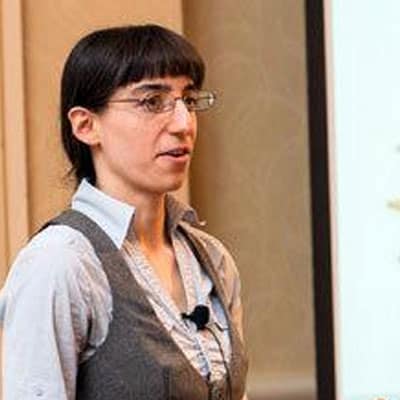
Brenda Rubenstein
Dr. Brenda Rubenstein is currently an Associate Professor of Chemistry and Physics at Brown University. While much of her group works on electronic structure theory, computational biophysics, and quantum computing, she is also deeply engaged in rethinking computing architectures. Prior to arriving at Brown, she was a Lawrence Distinguished Postdoctoral Fellow at Lawrence Livermore National Laboratory. She received her Sc.B.s in Chemical Physics and Applied Mathematics at Brown University, her M.Phil. in Computational Chemistry while a Churchill Scholar at the University of Cambridge, and her Ph.D. in Chemical Physics while a DOE Computational Science Graduate Fellow at Columbia University.
Summary:
As transistors near the size of molecules, computer engineers are increasingly finding themselves asking a once idle question: how can we compute using chemistry? In this talk, I will discuss recent progress my Brown Molecular Informatics team and I have made demonstrating how mixtures of small, unordered molecules can process information. During the first portion of this talk, I will illustrate how combinatorial chemical synthesis combined with high resolution mass spectrometry can be harnessed to store GBs of information in small molecules and metabolites. I will then turn to describing how basic principles of chemistry, such as mixing, complementarity, chemical reaction networks, and autocatalysis, can be exploited to realize fully molecular neural networks for machine learning and image processing. I will end with a discussion of the challenges molecular computation faces that may be resolved with clever doses of synthetic and theoretical chemistry, and the connections molecular computation has to the development of intelligent molecular machines.
By building a catalysis-driven motor into simulated numerical experiments, I systematically show how directionality responds when the motor is modified according to power-stroke intuition. Simulations confirm that the power stroke mechanism does not generally predict the directionality. Still, the relative stability of molecular conformations can nevertheless be a useful design element that helps one alter the directional bias of a molecular motor. The ostensible effectiveness of power-stroke intuition is explained by the recognition that to target conformation stability, one must alter interactions between moieties of a molecular motor, and those altered interactions do not affect the power stroke in isolation. This can lead to apparent correlations between power stroke and directionality that one might leverage when engineering specific systems.