Presenter
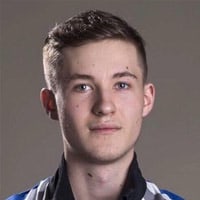
Dmitrii Usynin, Creator Fund
Dmitrii is a PhD student at a Joint Academy of Doctoral Studies (JADS) launched between Imperial College London and Technical University of Munich. His research interests lie in the domain of adversarial influence in collaborative machine learning, specializing in medical image analysis. Dmitrii is currently open for new collaborations on the topics of: Privacy attacks on AI systems, adversarial robustness of ML models, federated learning, differential privacy and collaborative learning for medical imaging. Dmitrii is also a privacy researcher at OpenMined, working on federated learning and differential privacy in healthcare. His recent works include “Zen and the art of model adaptation: Low-utility-cost attack mitigations in collaborative machine learning” (PETS 2022), “Adversarial interference and its mitigations in privacy-preserving collaborative machine learning” (Nature Machine Intelligence) and “End-to-end privacy preserving deep learning on multi-institutional medical imaging” (Nature Machine Intelligence).Dmitrii graduated from Imperial College London with an MEng in Computing and a distinguished project titled “Privacy-Preserving Machine Learning in a Medical Domain”.
Summary:
Machine learning (ML) relies on diverse and well-curated datasets, but obtaining them is challenging due to data protection regulations, low quality, and biases. Trustworthy Artificial Intelligence (TAI) addresses these issues with privacy-preserving, explainable, and fair model training. Privacy-preserving ML (PPML) ensures safe and robust AI systems. Challenges include scalable tools and incentives for participation. Approaches like differential privacy and homomorphic encryption can help secure distributed ML pipelines and protect privacy. This talk explores the state of PPML, its motivations, challenges, and necessary developments for broader adoption. Balancing privacy and ML model training is crucial. Overall, via ongoing research and development, Usynin aims to overcome the challenges surrounding PPML and foster the integration of privacy-enhancing techniques with other aspects of trustworthy AI.