Presenter
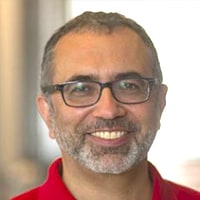
Mitya Chklovskii, Simons Foundation
The goal of Mitya Chklovskii’s research is to reverse engineer the brain on the algorithmic level. Informed by anatomical and physiological neuroscience data, his group develops algorithms that model brain computation and solve machine learning tasks. Chklovskii is also on the faculty of the NYU Medical Center. Before coming to the Simons Foundation in 2014, he was a group leader at Janelia Farm where he initiated and led a collaborative project that assembled the largest-at-the-time connectome, a comprehensive map of neural connections in the brain. Before that, he was an associate professor at Cold Spring Harbor Laboratory in New York, Sloan Fellow at the Salk Institute and Junior Fellow of the Harvard Society of Fellows. He holds a Ph.D. in physics from the Massachusetts Institute of Technology.
Summary:
In this talk, Mitya Chklovskii discusses the complexity of neurons and proposes a theoretical framework for understanding their function. He argues that the conventional view of neurons as feed-forward devices is oversimplified, and instead suggests that they should be considered as feedback controllers. This hypothesis eliminates the need for error backpropagation signals and allows neurons to learn from local information. Chklovskii introduces the direct data-driven control framework, which aligns with physiological observations and presents itself as a viable model for simulating neurons. He also discusses the challenge of inferring the degrees of freedom in neuronal dynamics and proposes learning the system’s parameters based on how well they can be learned. The speaker concludes by suggesting that neurons could implement a similar approach to adapt to unknown environments without needing a mechanistic model.