Presenter
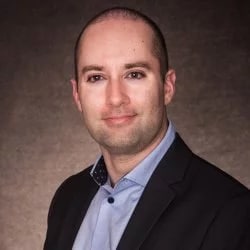
Roman Bauer, University of Surrey
I am a scientist in Computational Biology at the University of Surrey. After studying my basis year in Physics at ETH Zurich (Switzerland), I continued in Computational Science and Engineering (āRechnergestuetzte Wissenschaftenā) also at ETH Zurich. I then followed up with a PhD in Neuroinformatics, where I studied how neuronal networks develop and self-organise. I then made the life decision to go abroad and worked as a Postdoc and Research Fellow at Newcastle University (UK). Currently I am a lecturer at and lead and interdisciplinary research lab named āCOputational Modelling of Biological sYstems and engineeringā (COMBYNE) at the Department of Science at the University of Surrey.
Summary:
This talk summary by Roman Bauer focuses on modeling the structure of the brain and the challenges associated with it. He explains that while we are technologically capable of modeling and simulating the neural network at a human-scale level, the complexity of the brain with billions of neurons and trillions of synapses makes it challenging to create accurate models.
Bauer suggests leveraging the way nature has built the brain, starting from a single precursor cell and modeling how it generates biologically realistic neural tissues. By using a genetic approach, it becomes possible to scale up and create entire brains, allowing for a comparison with experimental data.
He also discusses the use of agent-based modeling, specifically a high-performance, open-source software called BioDynaMo, for modeling neural tissues. While currently not distributed, there is potential to implement large-scale, realistic brains using Python.
Bauer emphasizes the goal of moving towards personalized brain models by fine-tuning from a standardized blueprint brain. By including various experimental data, such as EEG, it becomes possible to create brain models that align with individual samples.
Overall, the talk highlights the challenges in modeling the brain structure, the potential of a genetic approach and agent-based modeling, and the importance of fine-tuning and personalization in creating accurate brain models.