Presenter
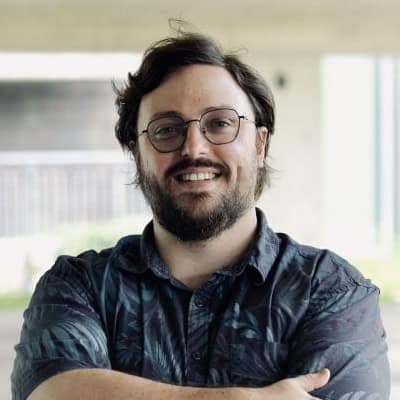
Tom Burns, SciAI Center - Cornell University
Tom Burns graduated with First Class Honours in his Bachelor of Science from Monash University in 2013, exploring the mathematical features of human perception of non-linguistic sounds. Shifting to philosophy, he completed a Master of Bioethics in 2014, analyzing the ethics of euthanasia legislation in Australia, followed by a World Health Organization Bioethics Fellowship in 2015, contributing to the Ebola epidemic response. As a Master of Philosophy by Research student, he focused on electrophysiological signals in the rat barrel cortex, concurrently holding leadership roles in neuroscience student organizations. The title of his PhD thesis at the Okinawa Institute of Science and Technology (OIST) in Japan was 'Geometry and Topology in Memory and Navigation,' wherein he employed ideas from higher mathematics to understand artificial and natural intelligence systems. In 2023, as a Fall Semester Postdoc at Brown University's ICERM, he contributed to the 'Math + Neuroscience' program. Recently affiliated with Timaeus, an AI safety organization, Tom is continuing his research at Cornell University's new SciAI Center from March 2024.
Summary:
Neuroscience is a burgeoning field with many opportunities for novel research directions. Due to experimental and physical limitations, however, theoretical progress relies on imperfect and incomplete information about the system. Artificial neural networks, for which perfect and complete information is possible, therefore offers those trained in the neurosciences an opportunity to study intelligence to a level of granularity which is beyond comparison to biological systems, while still relevant to them. Additionally, applying neuroscience methods, concepts, and theory to AI systems offers a relatively under-explored avenue to make headwind in the daunting challenges posed by AI safety — both for present-day risks, such as enshrining biases and spreading misinformation, and for future risks, including on existential scales. In this talk, I will present two emerging examples of interactions between neuroscience and AI safety. In the direction of ideas from neuroscience being useful for AI safety, I will demonstrate how associative memory has become a tool for interpretability of Transformer-based models. In the opposite direction, I will discuss how statistical learning theory and the developmental interpretability research program have applicability in understanding neuroscience phenomena, such as perceptual invariance and representational drift. These two examples motivate my current research in broadening and deepening the interface between these fields, especially in interpretability of biological and artificial neural networks.