Presenter
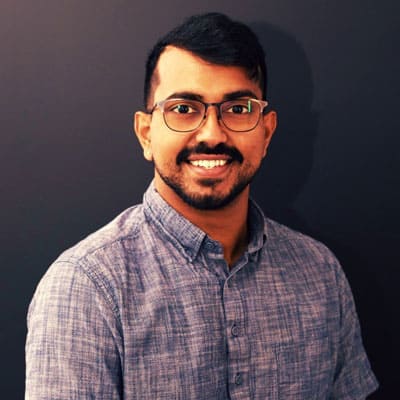
Varun Dwaraka, TruDiagnostic
Varun Dwaraka PhD is the Head of Bioinformatics at TruDiagnostic, and an aging and longevity investigator specializing in epigenetics and bioinformatics. He has co-authored numerous publications relating to genetics, epigenetic clocks, DNA methylation, and tissue regeneration. In 2020, Dr. Dwaraka was elected as a full member to the Sigma Xi, The Scientific Research Honor Society, and currently serves as a 2023 Foresight Fellow in Biotechnology and Health Expansion, awarded by the Foresight Institute. He also serves on the Scientific Advisory Board for SRW Labs based in New Zealand, offering insight into the application and interpretation of epigenetic age biomarkers. Dr. Dwaraka is passionate about implementing machine learning methods to advance predictive medicine, identify novel biomarkers, and create algorithms to better understand the biology of aging.
Summary:
Increasing age is the biggest risk factor for chronic disease and death, and therefore identifying ways to accurately predict biological age is imperative in understanding how interventions and therapeutics are affecting the aging process. In recent years, DNA methylation (DNAm) has shown remarkable associations to aging and longevity. However, these clocks have had issues which have presented problems with clinical application. Mainly, the precision of these markers has been limited, some clocks don’t respond to validated biological interventions, and immune cell changes have been confounding factors. Here we will present the previous issues with these clocks and how they have been solved with the first multi omi biological age score built using 75 clinical biomarkers, metabolomic data, proteomic data, and 60 + years of clinical EMR records. With the availability of additional -omic approaches, integrating DNAm based markers with additional markers of aging have provided the scientific standard for biological age quantification. This has culminated into a generation of a new generation of an OMIC clock, which exhibits higher associations to predicting higher incidence of disease but also shows significant predictive ability in predicting novel disorders (e.g., depression) which previously have not been correlated. We will discuss how this solution has fixed the previous issues and how it has found novel and exciting associations with age which can be applied clinically.