Presenters:
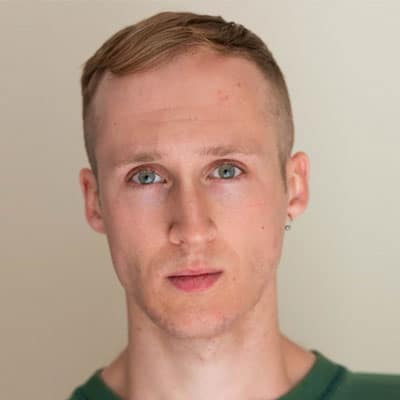
Lewis Hammond, Cooperative AI Foundation
My research concerns safety in multi-agent systems and in practice spans game theory, machine learning, and verification. One aspect of this that I spend a lot of time thinking about is how to make AI systems more cooperative, which is the mission of the Cooperative AI Foundation, where I work. At Oxford I am supervised by Michael Wooldridge, Alessandro Abate, and Julian Gutierrez, and am a DPhil Affiliate at the Future of Humanity Institute. I am also affilated with both the Centre for the Governance of AI and the Foresight Institute...
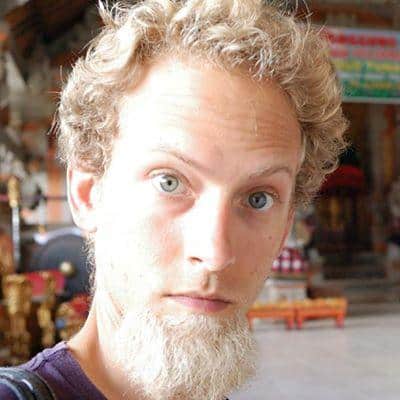
Keenan Pepper, Salesforce
Graduate student, University of California, Berkeley, physics department. Lead Software Engineer at Salesforce
Andrew Gritsevskiy, Cavendish Labs
I'm Andrew, a researcher who loves to learn about the world, make things, and go on adventures. I'm always excited to meet new people, so if you want to go hiking together, do a puzzle hunt, play a duet, or sneak into an oceanography conference, send me an email at [email protected]! My background is in deep reinforcement learning, complexity theory, and quantum information, though I am currently working on AI alignment. I am also quite interested in interdisciplinary approaches to neuroscience, drug development, and astronomy.
David Bloomin, Platypus AI
Specialties: c++, backends, databases, storage, scalable web services, machine learning, web frontend/backend development, java, python
Summary:
This working group focused on the concept of collusion between AI systems ā collusion here refers to cooperation between AI agents which negatively affects humans. They explored the use of communication channels like steganography or cryptography for collusion. The main objective was to detect and prevent collusion. The participants discussed viewing games or market designs as super agents formed by combining multiple agents and how this impacts collusion. They also examined game design parameters that make collusion easier or harder, such as private communication channels and persistent identity. They suggested surveying and categorizing different means of collusion, running simulations to understand mechanisms that lead to more collusion, and identifying ways to prevent those mechanisms. Potential downsides were mentioned, including the disruption of desired cooperation and the development of surveillance tools to detect collusion. The participants also discussed the challenge of understanding collusion at a small scale and the potential benefits of diversity among agents’ goals. Injecting agents into existing systems to monitor and promote competitive dynamics was proposed, as well as the idea of introducing nuance and turnover in the agent population to prevent collusion was mentioned. Overall, the group aimed to explore and prevent collusion in AI systems by analyzing game dynamics, mechanisms, and system design.
Ā